
01. How it Works
Integration of Data Sources
Initiating the auto-coding process, aiH.Automate™’s engine uses AI-ML to extract metadata from complex structured and unstructured clinical notes (FHIR, HL7/API, PDF, EMR/PM) to auto-populate demographic and clinical insights.
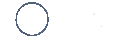
02. How it Works
Automated Coding Workflow
Next, the aiH.Automate™ engine assigns the correct procedural and diagnosis codes. Successfully coded notes are directly routed to billing workflows while complex exceptions are sent to human coders’ work queues for further review. QA sample rates can be customized by organization, provider, and specialty.
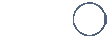
03. How it Works
Analytics and Full Audit Trailing
Our robust and customizable analytics dashboard offers full audit trailing, providing detailed insight into the auto-coding processes. Stakeholders can efficiently and transparently track the system’s performance every step of the way.
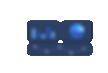
How it works.
aiH.Automate™ deploys advanced deep learning and AI-ML models to transform clinical notes into procedural and diagnosis codes. Our auto-coding engine sends completed encounters directly to billing while routing exceptions to coders for further review.
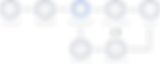
Reduce your coding workflow from minutes to seconds.
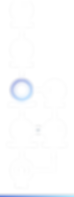
Reduce your coding workflow from minutes to seconds.
Reimagine Medical Coding with aiH.Automate™
AI-ML that dives deep into your medical specialties, extracting the clinical notes necessary to accurately and compliantly code for billing.

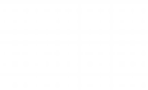
Why aiH.Automate™ is different from Computer Assisted Coding.
While CAC requires 100% human review and intervention, our self-learning platform automates 90+% of medical coding, reducing menial administrative burdens and increasing coding throughput by 50%.

Computer Assisted Coding
-
CAC requires 100% human review.
-
CAC does not adapt to the variability of unstructured provider documentation (e.g., clinical and/or operative notes) resulting in inaccurate coding suggestions.
-
Human intervention, re-work, missing documentation and denials impact revenue cycle and cost-to-collect performance.
-
Manual interventions impacts ability to scale and reduce cost of revenue cycle operations.
aiH.Automate™
-
aiH automates 90+% of medical coding routing complex exceptions to coders.
-
Self-learning AI-ML tools adapt to the variability of unstructured provider documentation, continuously improving coding accuracy, compliance, and clinical outcomes.
-
Reduced menial administrative burdens on staff and increased coding throughput by 50%.
-
Trustworthy AI-ML automates revenue cycle processes, addressing labor shortages while significantly decreasing charge lag by days.
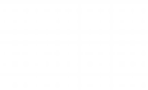